Online Learning to Overcome Catastrophic Forgetting on Non-Stationary Time Series Prediction
This work presents a practical approach for detecting non-stationarity in time series prediction. This method is called Spectral Evolution Analysis Feature Extraction (SAFE); it works by monitoring the evolution of the spectral contents of time series through a distance function.
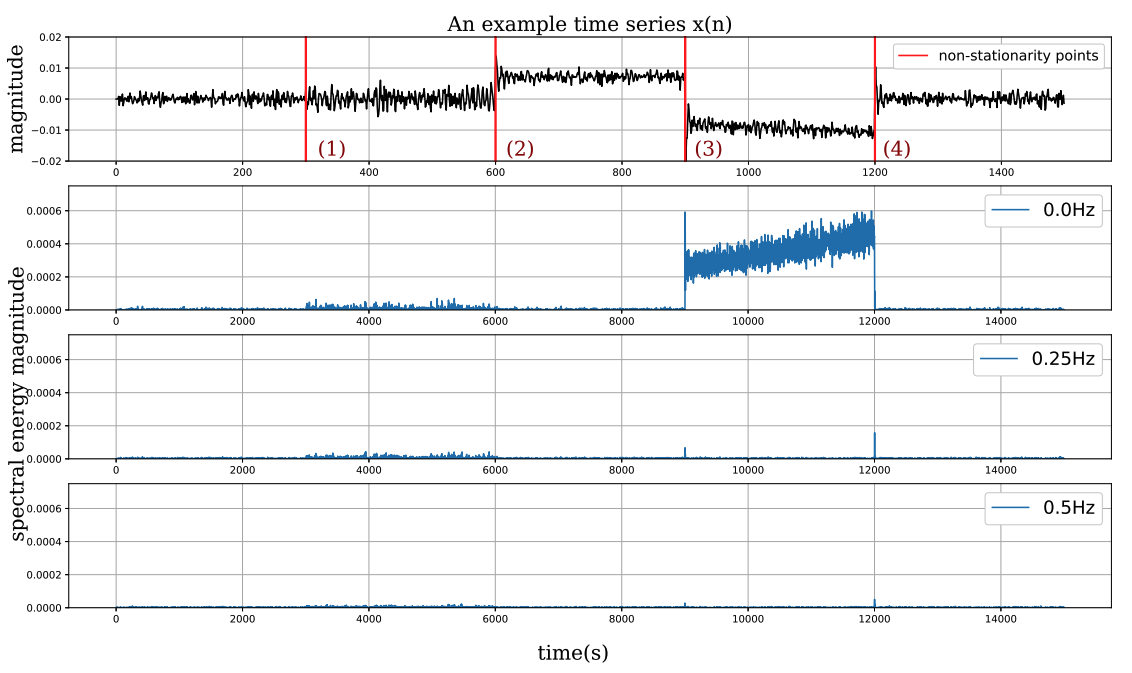
This method is designed to work in combination with state-of-the-art machine learning methods in real time by informing the online predictors to perform necessary adaptation when a non-stationarity presents. We also propose an algorithm to proportionally include some past data in the adaption process to overcome the Catastrophic Forgetting problem.
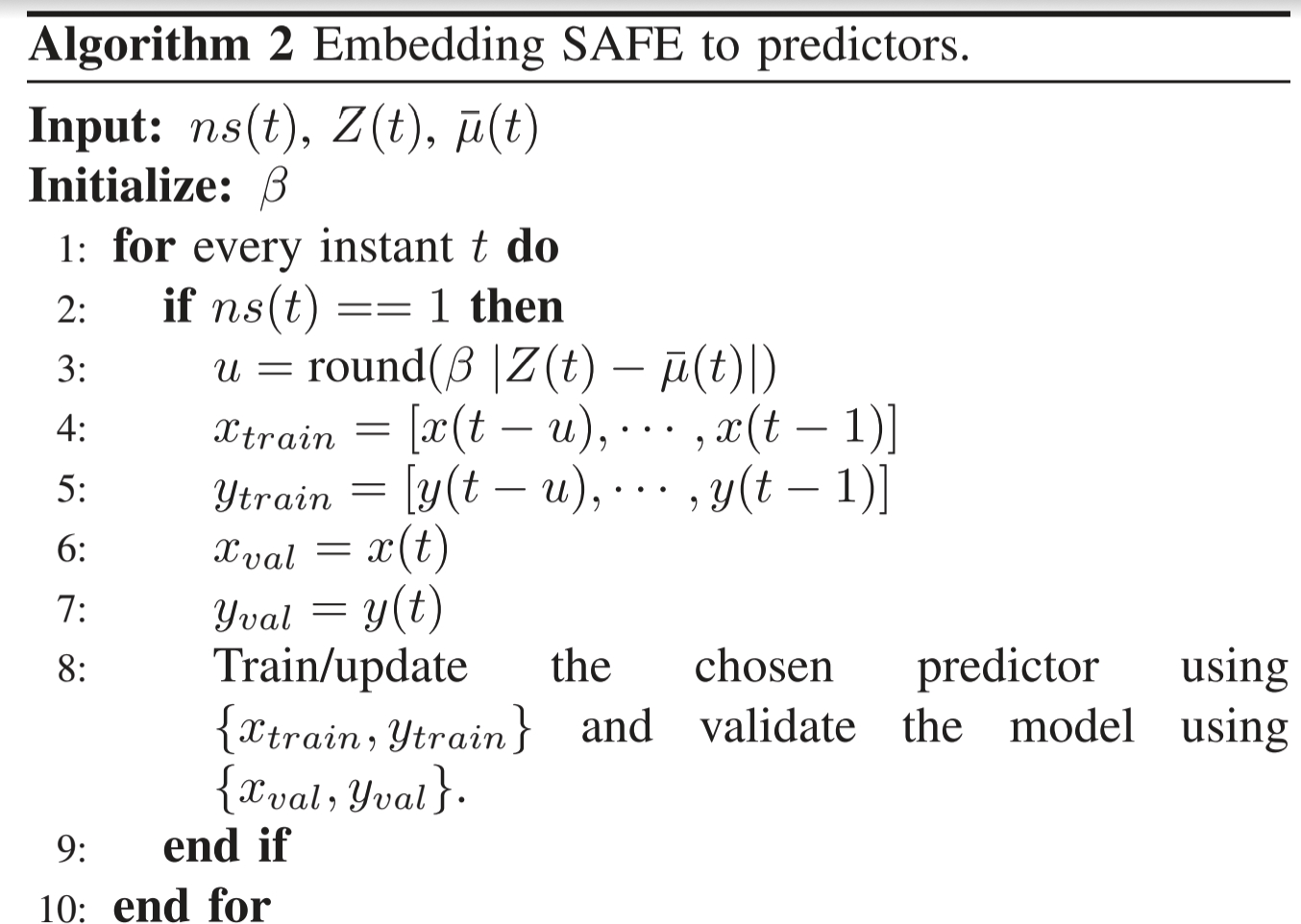
To validate our hypothesis and test the effectiveness of our approach, we present comprehensive experiments in different elements of the approach involving artificial and real-world datasets. The experiments show that the proposed method is able to significantly save computational resources in term of processor or GPU cycles while maintaining high prediction performances.
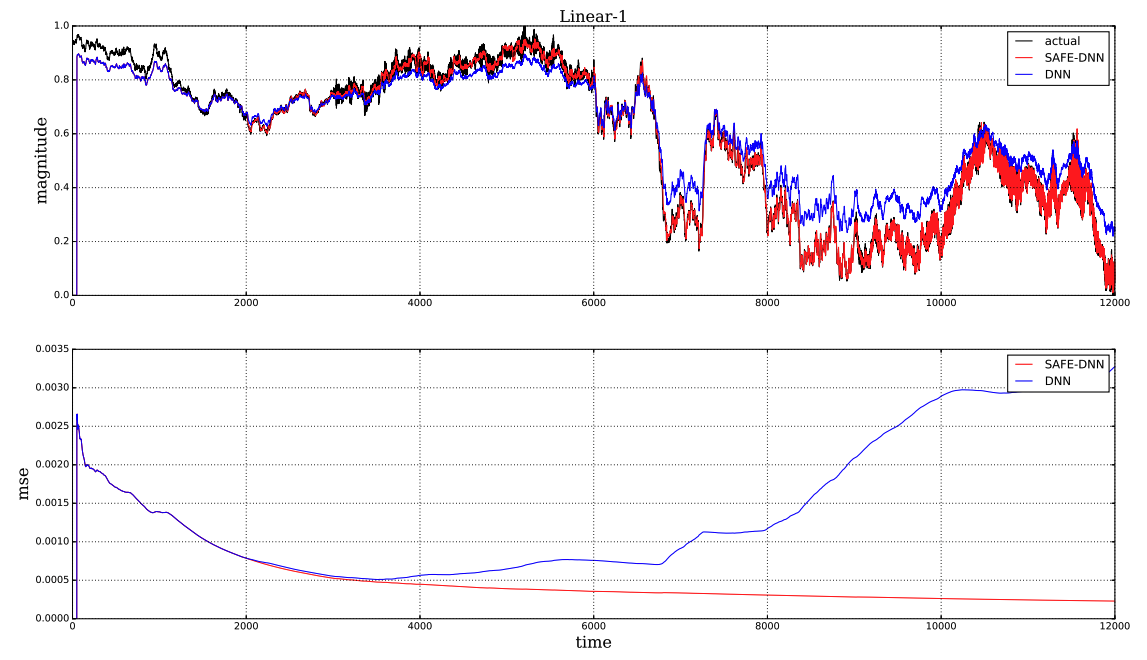
N.B. The details of the work can be seen in this paper: Arief Koesdwiady, and Fakhry Karray. “SAFE: Spectral Evolution Analysis Feature Extraction for Non-Stationary Time Series Prediction”. arXiv preprint arXiv:1803.01364 (2018). [link]